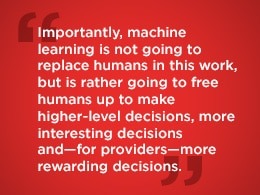
Stephen Traub, MD is currently in his eighth year as Chairman of a major Phoenix, Arizona Emergency Department. During his career, he’s witnessed numerous healthcare technology deployments, and accrued key insights into implementation strategies that drive value. As part of an ongoing series about practical applications for Artificial Intelligence in healthcare, we asked Dr. Traub, an advisor to Qventus, for his perspective on some of the challenges facing hospitals today as they explore new technologies that have the potential to improve the staff and patient experience.
1. What technology trends do you feel have had the most impact on delivering care in the ED?
Doctor Stephen Traub: Adoption of electronic medical records (EMRs), which has been a double-edged sword. Although the data from these systems has given many of us the ability to learn more about our operations, EMRs have come at a high cost. EMRs, at least in my experience, have been clunky and have negatively impacted our efficiency and productivity.
2. What’s the biggest challenge for deploying technology to support healthcare?
ST: A lot of the challenge in deployment is adoption of new practices and sustained change management. Even when things are difficult, there’s something of a devil-you-know versus devil-you-don’t-know approach to technology. I think that’s only been exacerbated by the fact that EMRs, which were sold as something that would help our productivity, actually interfered with it. To some extent EMRs have gotten in the way of our taking care of patients in a compassionate and efficient manner. I think that the emergency medicine community in particular has a bit of negative bias when it comes to using technology to improve what we do because many felt the initial promise of EMRs didn’t pan out. I think you have to overcome that initial skepticism.
3. What technologies are you seeing now with the promise of helping you extract value from EMRs?
ST: Any product or any program that a hospital can use in the ED to extract granular visit-level data from its EMR has tremendous promise to improve operations. We have the ability, through a couple of different mechanisms, to do this which allows me to look at specific examples of the sort of problems we face. What throughput metrics can I see for individual providers, be they doctors or nurses? What kind of throughput metrics do we see with respect to admission to different services? How are providers achieving the performance goals that we set for them? Being able to see this data gives me robust and accurate feedback to different providers or different services, so we can all try to manage in the right direction with respect to performance. That’s what we’re doing on a day-to-day and week-to-week basis.
However, I think that the ultimate goal for us, in the ED, is to use our data even more aggressively and in a more timely manner. This includes making operational changes in real time to keep the department moving, and also leveraging this data, through machine learning, to discern the most important associations regarding operations. Humans just can’t process data to the same extent and speed that computer-based machine learning can.
4. A recent study from the Academy said that less than 39 percent of hospital executives are looking at or considering artificial intelligence solutions. Is that because they don’t realize A.I. and machine learning are actually driving the solutions they’re considering and/or deploying?
ST: What I can tell you is that I personally believe 39 percent is a relatively robust number. Remember that, up until several years ago, A.I. really had not entered the mainstream discussion in terms of healthcare solutions. So, the fact that you have this degree of buy-in is a big step forward. I can also tell you that I would be one of those people in the 39 percent who believes that machine learning and A.I. will open up great doors for us in terms of our productivity.
5. Where do you see the potential for A.I. in healthcare?
ST: I can give you an example. In the ED, one of the things that we look at is patients who return within 72 hours. Many of us look at that as a potential opportunity for improvement. Currently, we don’t really have great predictors as to who will come back. My feeling, my suspicion and my hope is that A.I. and machine learning will help us use inputs of multiple variables – more variables than any one human being could ever process – to help us understand the profile of patients discharged from the ED who are more likely to return. This could give us the ability to intervene at the time of discharge, so that we can try to make sure we answer questions and reduce the number of patients who come back.
6. What would you say to your peers when considering or deploying an A.I. application?
ST: Make sure that the machine-learning program is going to give you the outputs that you want. The key to this is collaboration with the machine-learning group (vendor or in-house developer) you’re working with. A software engineer left to his or her own devices may not come up with outcomes that are as clinically important as the outcomes that you believe are important. Close partnerships between clinicians, administrators and those who are programming the machine-learning solution are really important to success.
I also think that the application that frees up a physician, nurse or charge nurse in the ED to make higher-level decisions is the application that is most likely to succeed. The winning application is one that performs a routine analysis of data and then gives direction, freeing up the person who would otherwise have to scan some electronic white board or tracking board to come up with a conclusion himself or herself. That would allow the care provider to focus on the patient, rather than hunting down a lab result or open bed.
6. You mentioned the “false promise,” so to speak, of EMRs. Do you have any use cases about how A.I. has made analysis of EMR more efficient or productive?
ST: The short answer is no. Not yet. But I think that the more interesting – and more important – answer is that we’re just at the dawn of using machine learning to assist us in healthcare. So, while I can’t give you a lot of use cases now, I think that if you were to ask me this question even 18 months or two years from now you’ll get a very different answer.
7. Any other insights you’d like to share with us that you’d want your peers to know about?
ST: There is little, that I’ve seen in the last several years, that has the ability to improve our productivity and our accuracy to the extent that machine learning does. Much of the thinking about patients and how to make diagnoses and move them through complex operating environments can probably be done faster and perhaps even more accurately through machine-learning algorithms. Importantly, machine learning is not going to replace humans in this work, but is rather going to free humans up to make higher-level decisions, more interesting decisions and–for providers–more rewarding decisions.